Agentic Swarm
Exponential instance-based work multiplier
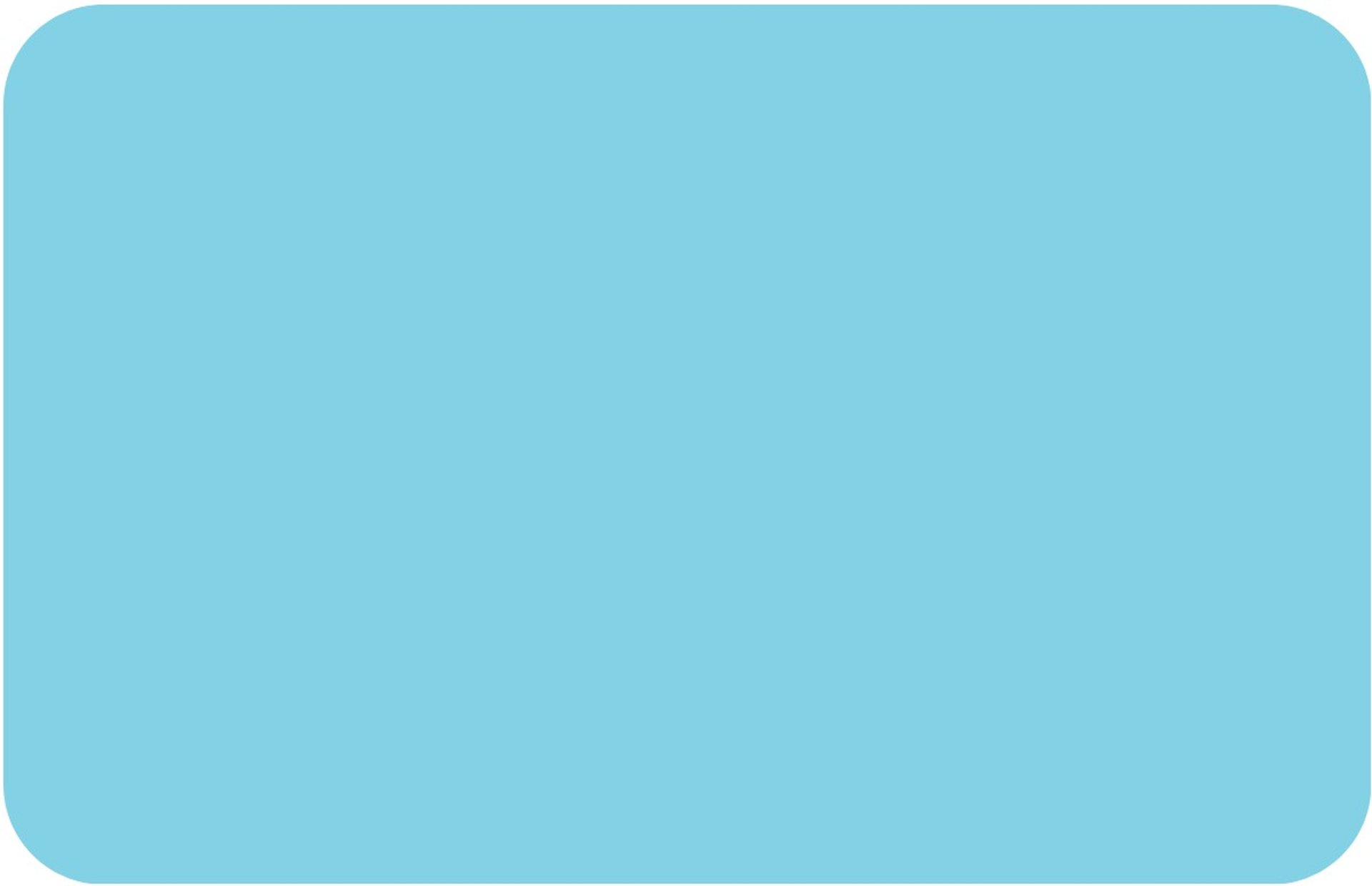
Intra-minute inference and action paths
Agentic swarms, composed of decentralized AI agents operating in concert, are emerging as a powerful paradigm for intra-minute cryptocurrency trading. Unlike traditional algorithmic trading systems that rely on centralized logic, agentic swarms distribute decision-making across a network of autonomous agents, each with specialized roles—such as signal detection, sentiment analysis, arbitrage identification, and risk assessment. These agents continuously communicate and adapt to volatile market conditions, mimicking swarm intelligence observed in nature.
In intra-minute trading, where price movements occur within seconds, the speed and coordination of agentic swarms offer a competitive edge. They analyze multiple data streams in parallel, including order books, social media sentiment, transaction patterns, and technical indicators, to detect micro-opportunities that human traders or slower bots would miss. Once a profitable condition is detected, the swarm can rapidly allocate capital and execute trades with minimal latency.
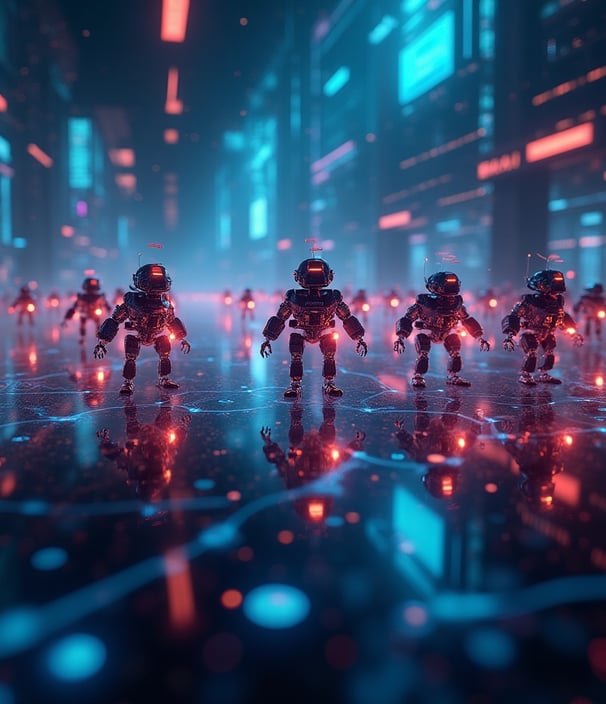
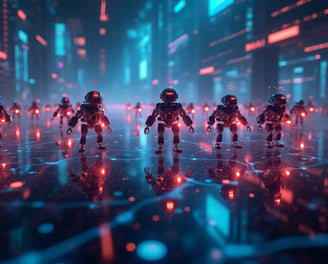
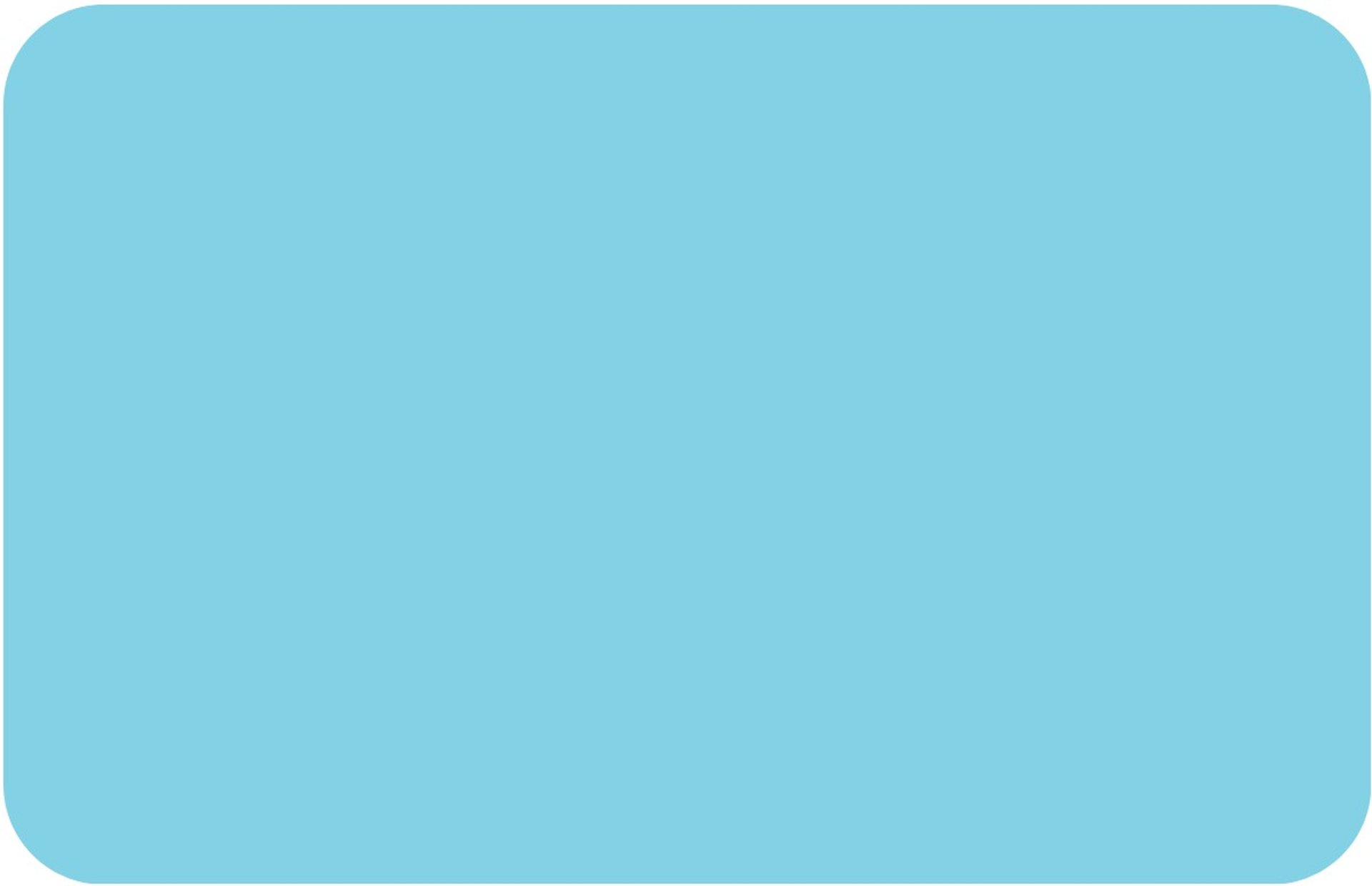
Chain-of-work via agents and Web3 arbitrage
Creating social network graph subsets from popular social media platforms is a powerful method for simulating and understanding consumer behavior patterns in a digitally connected world. Social networks like Instagram, Twitter, Facebook, TikTok, and Reddit are rich with structured and unstructured interaction data—including follows, likes, comments, shares, and messaging—that can be modeled as graphs. In these graphs, users are nodes, and interactions or relationships between them form the edges. Subsetting these graphs—through sampling, community detection, or interest-based clustering—enables researchers and marketers to create manageable, focused datasets that preserve real-world dynamics while maintaining privacy and scalability.
By isolating subsets tied to specific behaviors (e.g., frequent buyers, trendsetters, brand advocates), brands can study diffusion of influence, opinion formation, and purchasing decisions in micro-environments. Graph algorithms like PageRank, centrality measures, and clustering coefficients help identify key influencers, subcommunities, and pathways of information flow. These insights can be used to simulate scenarios such as viral marketing, targeted advertising, and customer churn prediction.
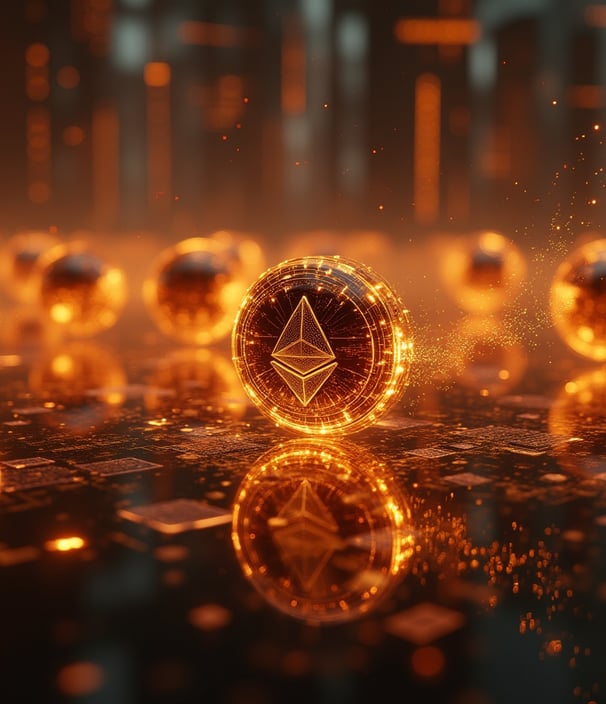
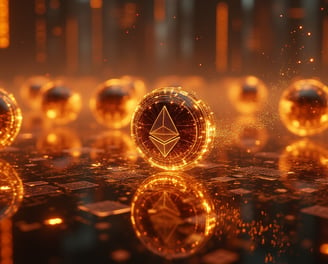